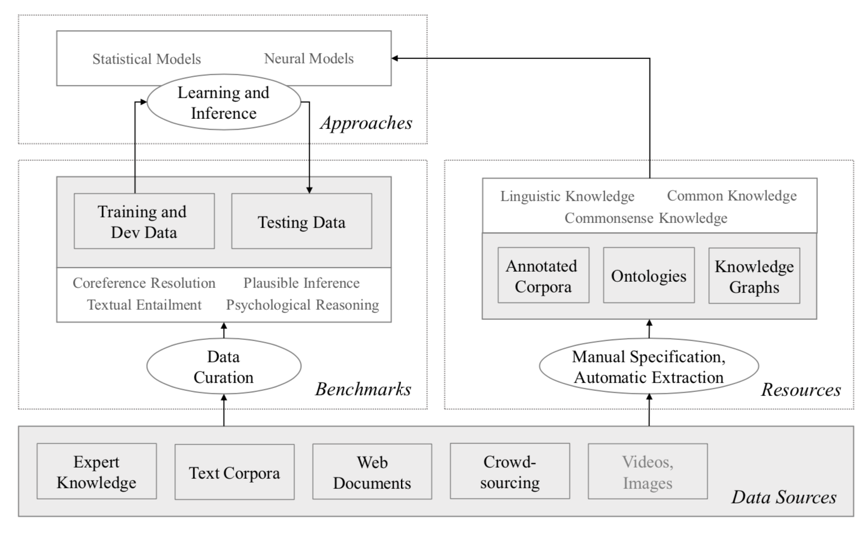
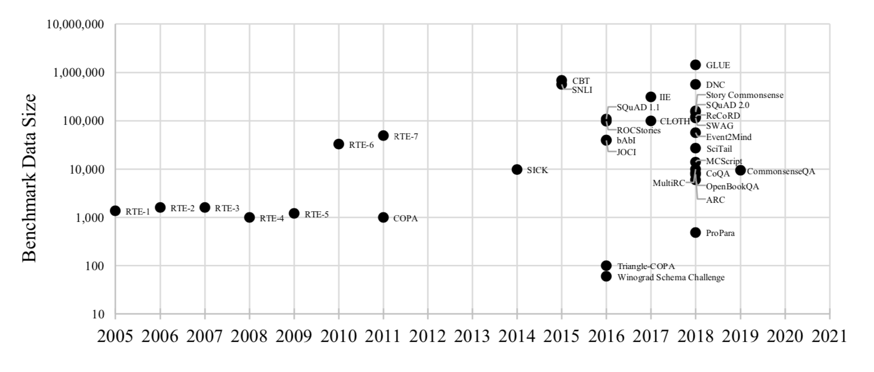
1. CATEGORIZING COMMONSENSE
First of all, let's make it clear that there can be many kinds of commonsense! Here I categorize commonsense according to the training strategies and inductive biases.
1. Implicit knowledge
o Knowledge behind text and images.
o Example: “Everyone knows that ...”
2. Explicit knowledge (Memory Capacity)
o Knowledge in Wikipedia, knowledge in long stories.
o Example: “Not everyone knows it! You learn it only when you read the Wikipedia page or learn it from books.” == “only appears once (or few times) in the whole dataset!”
3. Reasoning Capacity
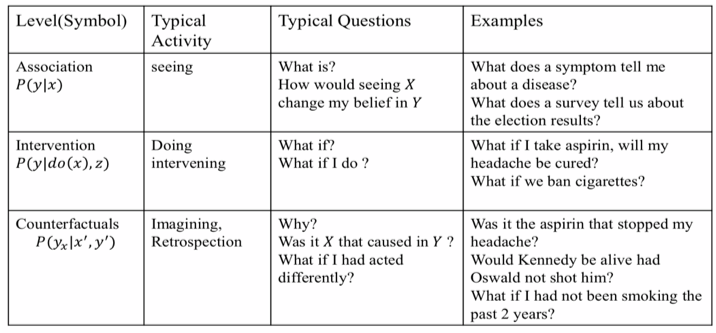
You cannot expect BERT/GPT to do intervention-level reasoning or counterfactual reasoning because they are just statistical models. But for implicit directional relationships which I believe belong to “implicit knowledge”, LM should have the capability to capture them.
2 LEARNING AND INFERENCE APPROACHES
2.1. INCORPORATING EXTERNAL KNOWLEDGE
It is worth noting that what kind of commonsense is incorporated into neural models depends on what kind of knowledge resource is used in one specific work. I think the key feature of this approach is that there exist some kinds of “knowledge embeddings” which can be concatenated (or use other technics) to original embeddings.

2.2. MEMORY AUGMENTATION:
MemNet, EntNet, KG-MRC.
· Using memory components
· Targeted the tasks which require “memory capability”, such as bAbI, CBT, Propara.
2.3. COMMONSENSE CAUSAL REASONING
This line of work has developed from statistical approaches (such as PMI) towards neural approaches. I would rather consider them as approaches to learn “causal implicit knowledge” instead of reasoning. It is worth noting that some work does try to separate necessity causality and sufficiency causality.
Comments